Slide 1 of 3 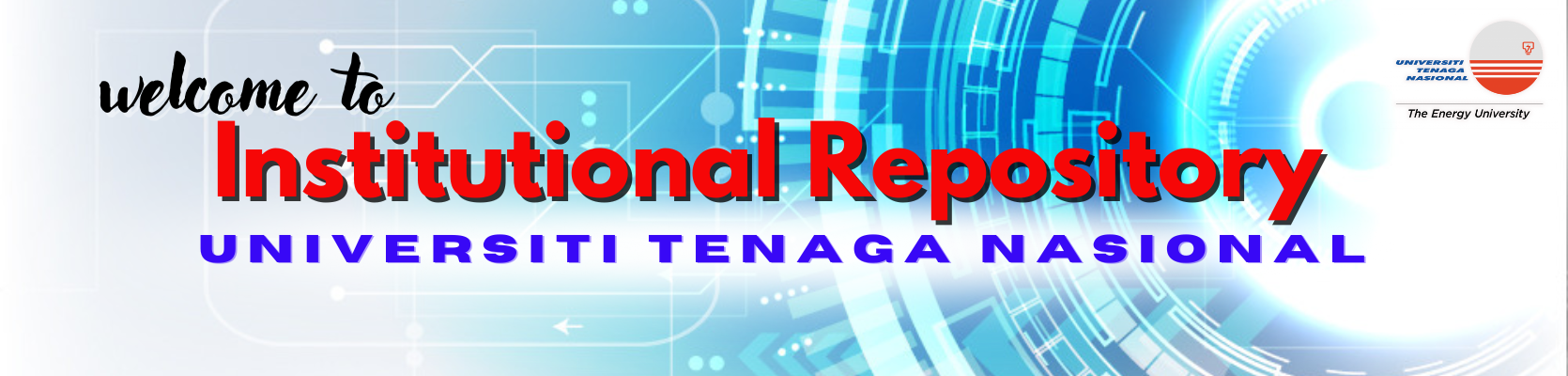
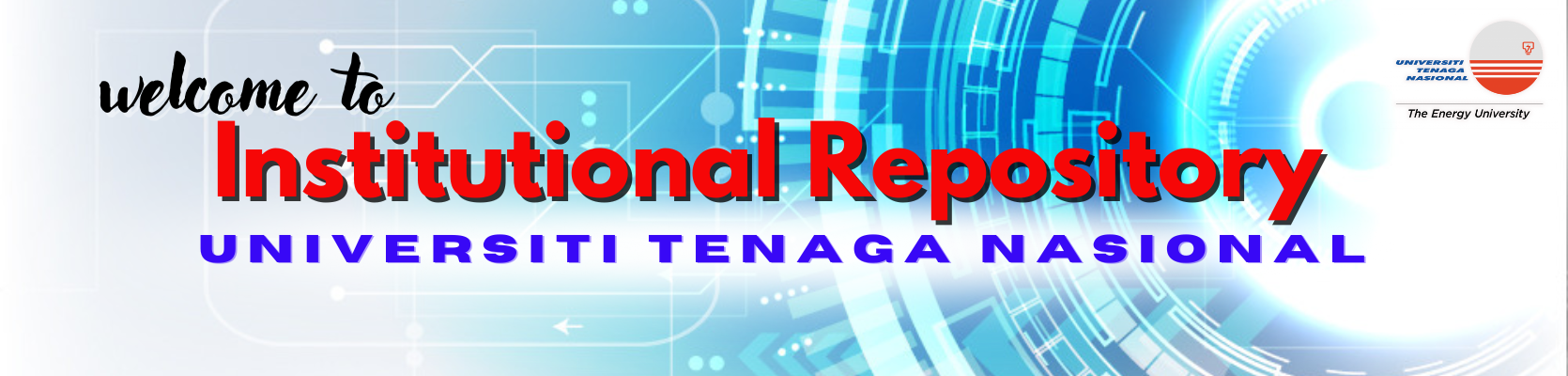
Slide 2 of 3 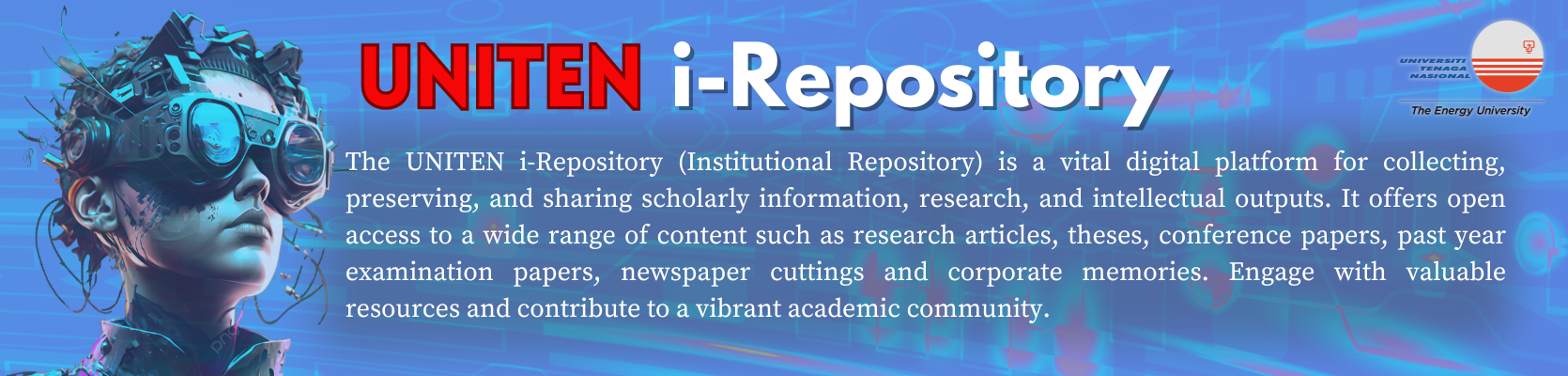
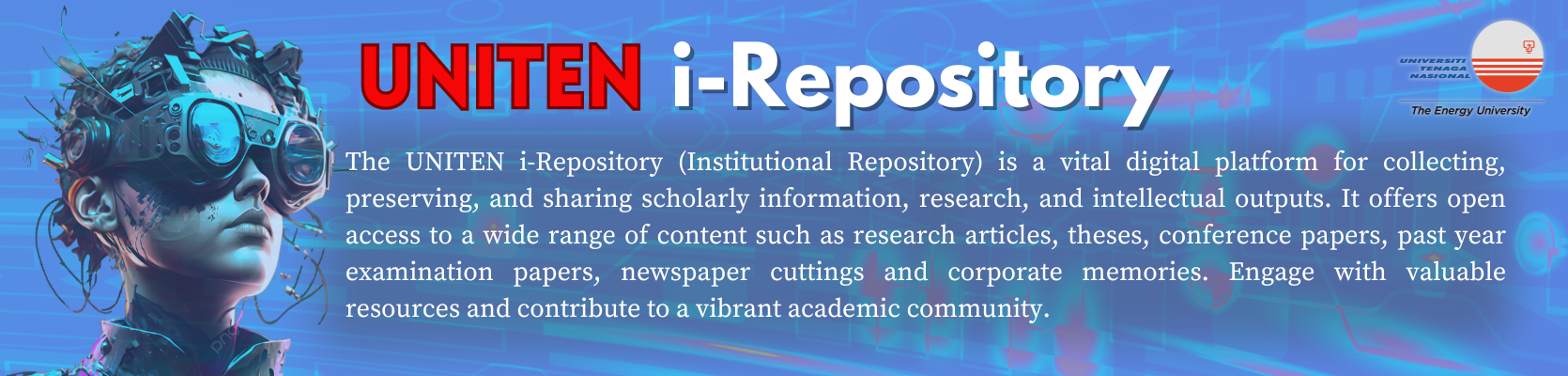
Slide 3 of 3 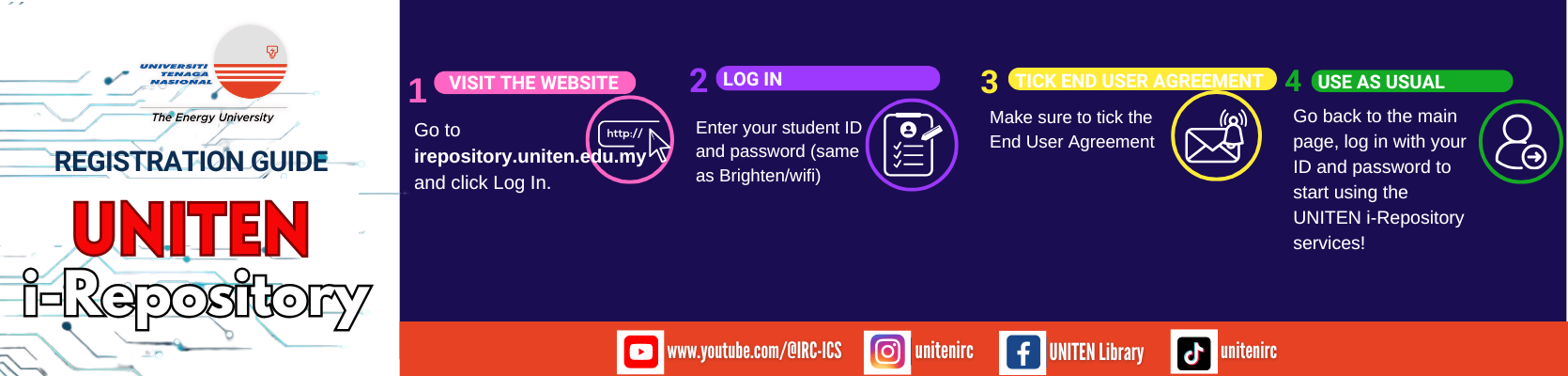
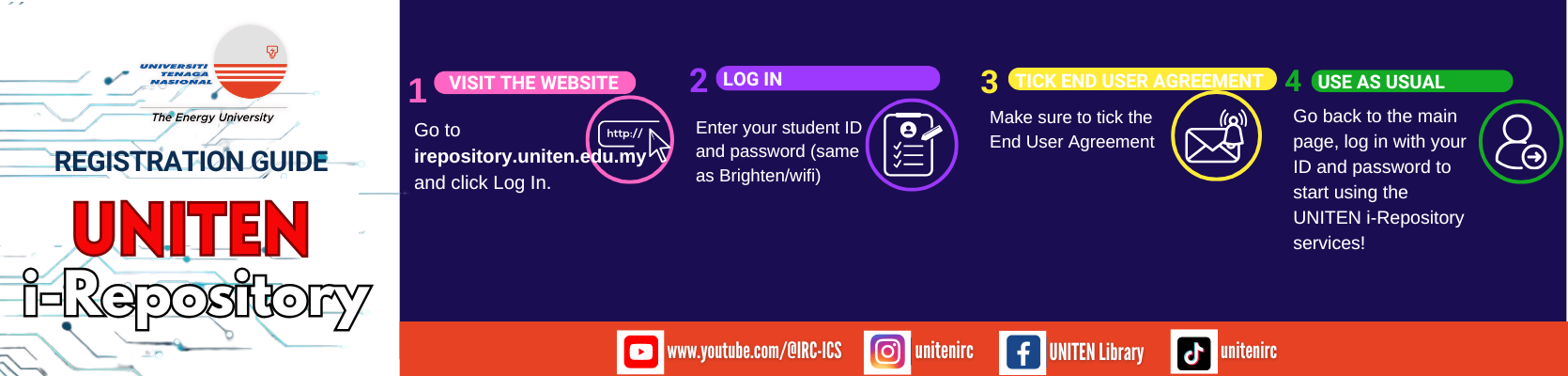
Research outputs
28490
Projects
0
People
3
Recent Additions
- PublicationCalculus 3 - MATB213 - Semester November, 1999/2000(2025-06-25)
- Publication
- Publication
- Publication
- Publication
Most viewed
- Publication
- Publication
- Publication